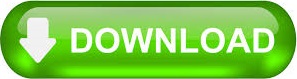

In the section, Procedure, we illustrate the SPSS Statistics procedure to perform a point-biserial correlation assuming that no assumptions have been violated. Just remember that if you do not run the statistical tests on these assumptions correctly, the results you get when running a point-biserial correlation might not be valid. You can check assumptions #3, #4 and #5 using SPSS Statistics. You can test this using Levene's test of equality of variances.
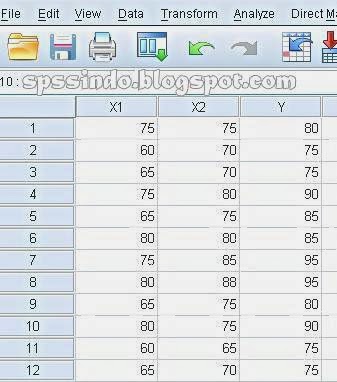
#KORELASI SERIAL DENGAN SPSS HOW TO#
This is not uncommon when working with real-world data rather than textbook examples, which often only show you how to carry out a point-biserial correlation when everything goes well! However, don’t worry.

In practice, checking for these five assumptions just adds a little bit more time to your analysis, requiring you to click a few more buttons in SPSS Statistics when performing your analysis, as well as think a little bit more about your data, but it is not a difficult task.īefore we introduce you to these five assumptions, do not be surprised if, when analysing your own data using SPSS Statistics, one or more of these assumptions is violated (i.e., is not met). You need to do this because it is only appropriate to use a point-biserial correlation if your data "passes" five assumptions that are required for a point-biserial correlation to give you a valid result. When you choose to analyse your data using a point-biserial correlation, part of the process involves checking to make sure that the data you want to analyse can actually be analysed using a point-biserial correlation. However, before we introduce you to this procedure, you need to understand the different assumptions that your data must meet in order for a point-biserial correlation to give you a valid result. This "quick start" guide shows you how to carry out a point-biserial correlation using SPSS Statistics, as well as how to interpret and report the results from this test. Alternately, you could use a point-biserial correlation to determine whether there is an association between cholesterol concentration, measured in mmol/L, and smoking status (i.e., your continuous variable would be "cholesterol concentration", a marker of heart disease, and your dichotomous variable would be "smoking status", which has two categories: "smoker" and "non-smoker").
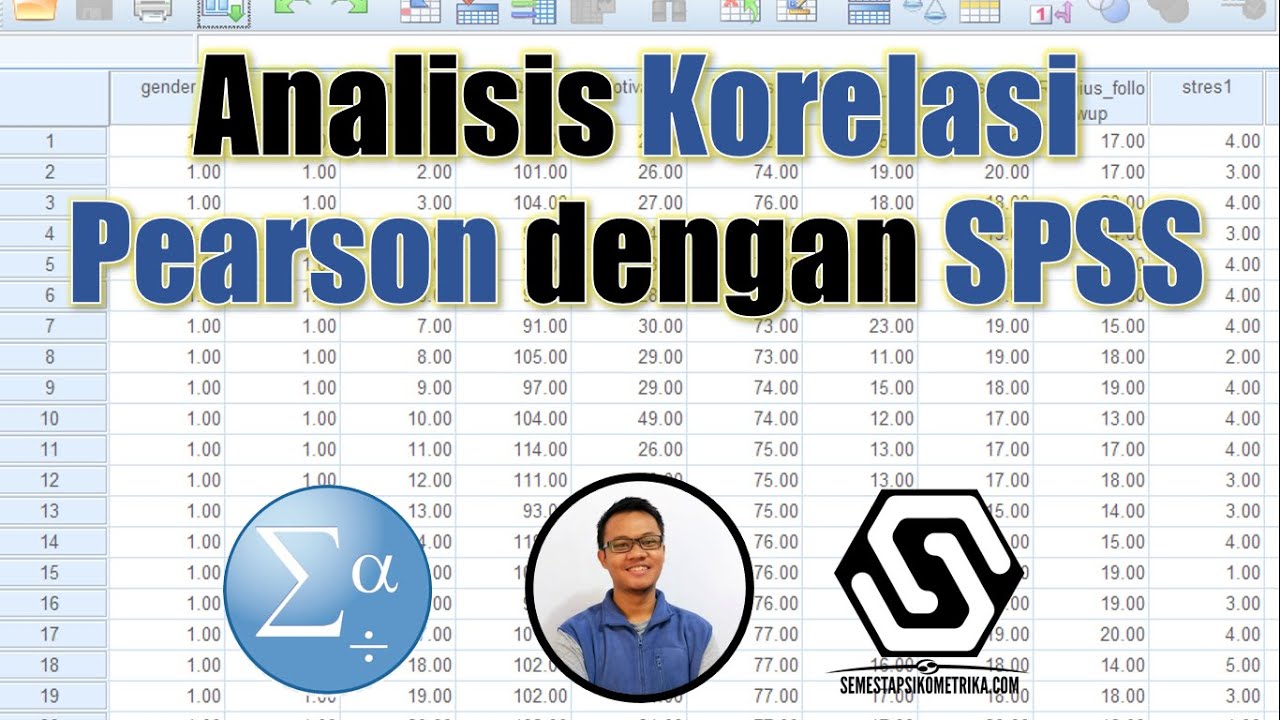
It is a special case of the Pearson’s product-moment correlation, which is applied when you have two continuous variables, whereas in this case one of the variables is measured on a dichotomous scale.įor example, you could use a point-biserial correlation to determine whether there is an association between salaries, measured in US dollars, and gender (i.e., your continuous variable would be "salary" and your dichotomous variable would be "gender", which has two categories: "males" and "females"). Point-Biserial Correlation using SPSS Statistics IntroductionĪ point-biserial correlation is used to measure the strength and direction of the association that exists between one continuous variable and one dichotomous variable.
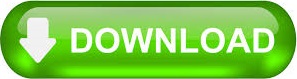